Research on distributed systems is often motivated by some variation of the following:
Developers of distributed systems face notoriously difficult challenges, such as concurrency, asynchrony, and partial failure.
That statement seems convincing enough, but it’s rather abstract. In this post
we’ll gain a concrete understanding of what makes distribution so challenging,
by describing correctness bugs we found in an implementation of the Raft consensus protocol.
Raft is an interesting example because its authors designed it to be
understandable and straightforward to implement. As we’ll see, implementing even the relatively
straightforward Raft spec correctly requires developers to deal with many difficult-to-anticipate
issues.
Fuzz testing setup
To find bugs we’re going to employ fuzz testing. Fuzz tests are nice because
they help us exercise situations that developers don’t anticipate
with unit or integration tests. In a distributed environment,
semi-automated testing techniques such as fuzzing are especially useful, since the number of possible
event orderings a system might encounter grows exponentially with the number
of events (e.g. failures, message deliveries)–far too many cases for developers to reasonably cover with
hand-written tests.
Fuzz testing generally requires two ingredients:
- Assertions to check.
- A specification of what inputs the fuzzer should inject into the system.
Assertions
The Raft protocol already has a set of nicely defined safety
conditions, which we’ll use as our assertions.
Figure 3 from the Raft paper (copied left) shows Raft's key invariants.
We use these invariants as our assertions. Each assertion should hold at
any point in Raft's execution.
For good measure, we also add in one additional assertion:
no Raft process should crash due to an uncaught exception.
We'll check these invariants by periodically halting the fuzz test and
inspecting the internal state of each Raft process. If any of the assertions
ever fails, we've found a bug.
The trickier part is specifying what inputs the fuzzer should inject.
Generally speaking, inputs are anything processed by the system, yet created outside the control of
the system. In the case of distributed systems there are a few sources of
inputs:
- The network determines when messages are delivered.
- Hardware may fail, and processes may (re)join the system at random points.
- Processes outside the system (e.g. clients) may send messages
to processes within the system.
To generate the last two types of inputs, we specify a function for creating random external messages (in the case of Raft: client commands)
as well as probabilities for how often each event type (external message sends, failures, recoveries) should be injected.
We gain control over the network by interposing on the distributed system’s RPC layer, using AspectJ. For now, we target a
specific RPC system: Akka. Akka is ideal because it provides
a narrow, general API that operates at a high level abstraction
based around the actor model.
Our interposition essentially allows us to play god: we get to choose exactly when each
RPC message sent by the distributed system is delivered. We can delay, reorder, or drop any message the
distributed system tries to send. The basic architecture of our test harness
(which we call DEMi) is shown below:
Every time a process sends an RPC message, the Test Harness intercepts it and places
it into a buffer. The Test Coordinator later decides when to deliver that message to the recipient.
In a fully asynchronous network, the Test Coordinator can arbitrarily delay
and reorder messages.
The Test Coordinator also injects external events (external message sends, failures, recoveries)
at random according to the probability weights given by the fuzz test
specification.
Interposing at the RPC layer has a few advantages over interposing at a
lower layer (e.g. the network layer, a la Jepsen).
Most importantly, we get fine-grained control over when each individual
(non-segmented) message is delivered. In contrast, iptables is a much more blunt tool: it only allow the tester
to drop or delay all packets between a given pair of processes [1].
Targeting applications built on Akka gives us one other
advantage: Akka provides a timer API that obviates the need for application
developers to read directly from the system clock. Timers are a crucial part of distributed systems,
since they are used to detect failures. In Akka, timers are modeled
as messages, to be delivered to the process that set the timer at a later
point in the execution. Rather than waiting for the wall-clock time for each
timer to expire, we can deliver it right away, without the application
noticing any difference.
Target implementation: akka-raft
The Raft implementation we target is akka-raft. akka-raft is written by one of the
core Akka developers, Konrad Malawski. akka-raft is fully featured according to
the Raft implementation page; it supports log
replication, membership changes, and log compaction. akka-raft has existing
unit and integration tests, but it has not yet been deployed in production.
UPDATE: Konrad asked me to include a short note, and I’m glad to oblige:
akka-raft is not an officially supported Akka module, but rather just a side project of Konrad’s. The Akka modules themselves are much more rigorously tested before release.
For our fuzz tests we set up a small 4-node cluster (quorum size=3). akka-raft
uses TCP as its default transport protocol, so we
configure DEMi to deliver
pending messages one-at-a-time in a semi-random order that obeys FIFO order
between any pair of processes. We also tell DEMi to inject a given number of client
commands (as external messages placed into the pending message buffer), and
check the Raft invariants at a fixed interval throughout the execution. We do
not yet exercise auxiliary features of akka-raft, such as log compaction or
cluster membership changes.
Bug we found
For all of the bugs we found below, we first minimized the faulty execution
before debugging the root cause [2]. With the minimized execution in hand,
we walked through the sequence of message deliveries in the
minimized execution one at
a time, noting the current state of the process receiving the message. Based on
our knowledge of the way Raft is supposed to work, we found the places in
the execution that deviated from our understanding of correct behavior.
We then examined the akka-raft code to understand why it deviated, and came up
with a fix. We submitted all of our fixes as pull requests.
A few of these root causes had already been pointed out
by Jonathan Schuster through a manual audit of the code, but
none of them had been verified with tests or fixed before we ran our fuzz
tests.
On with the results!
raft-45: Candidates accept duplicate votes from the same election term.
Raft is specified as a state machine with three states: Follower
, Candidate
,
and Leader
. Candidates attempt to get themselves elected as leader by
soliciting a quorum of votes from their peers in a given election term (epoch).
In one of our early fuzz runs, we found a violation of ‘Leader Safety’, i.e. two
processes believed they were leader in the same election term. This is a bad
situation for Raft to be in, since the leaders may overwrite each other’s log
entries, thereby violating the key linearizability guarantee that Raft is supposed to
provide.
The root cause here was that akka-raft’s candidate state did not detect
duplicate votes from the same follower in the same election term.
(A follower might resend votes because it believed that an earlier vote was
dropped by the network). Upon receiving the duplicate vote, the candidate
counts it as a new vote and steps up to leader before it actually
achieved a quorum of votes.
raft-46: Processes neglect to ignore certain votes from previous terms.
After fixing the previous bug, we found another execution where two leaders
were elected in the same term.
In Raft, processes attach an ‘election term’ number to all messages they send.
Receiving processes are supposed to ignore any messages that contain an
election term that is lower than what they believe is the current term.
akka-raft properly ignored lagging term numbers for some, but not all message
types. DEMi delayed the delivery of messages from previous
terms and uncovered a case where a candidate incorrectly accepted a vote message from
a previous election term.
raft-56: Processes forget who they voted for.
akka-raft is written as an FSM. When
making a state transition, FSM processes specify both which state
they want to transition to, and which instance variables they want to keep
once they have transitioned.
All of the state transitions for akka-raft were correct except
one: when a candidate
steps down to follower (e.g., because it receives an AppendEntries
message,
indicating that there is another leader in the cluster), it
forgets which process it previously voted for in that term. Now, when another
process requests a vote from it in the same term, it will vote again but this
time for a different process than it previously voted for, allowing
two leaders to be elected.
raft-58a: Pending client commands delivered before initialization occurs.
After ironing out leader election issues, we started finding other issues. In
one of our fuzz runs, we found that a leader process threw an assertion error.
When an akka-raft candidate first makes the state transition to leader, it does not
immediately initialize its state (the nextIndex
and matchIndex
variables).
It instead sends a message to itself,
and initializes its state when it receives that self-message.
Through fuzz testing, we found that it is possible that the candidate could have pending ClientCommand
messages
in its mailbox, placed there before the candidate transitioned to leader
and sent itself the initialization message.
Once in the leader state, the Akka runtime will first deliver the ClientCommand
message. Upon processing the ClientCommand
message the leader tries to replicate it to the rest of the cluster, and updates its
nextIndex
hashmap.
Next, when the Akka runtime delivers the initialization self-message, it will overwrite the
value of nextIndex
. When it reads from nextIndex
later, it’s possible for
it to throw an assertion error because the nextIndex
values are
inconcistent with the contents of the leader’s log.
raft-58b: Ambiguous log indexing.
In one of our fuzz tests, we found a case where the ‘Log Matching’
invariant was violated, i.e. log entries did not appear in the same order on
all machines.
According to the Raft paper, followers should reject AppendEntries
requests from leaders that are
behind, i.e. prevLogIndex
and prevLogTerm
for the AppendEntries
message are
behind what the follower has
in its log. The leader should continue decrementing its nextIndex
hashmap
until the followers stop rejecting its AppendEntries
attempts.
This should have happened in akka-raft too, except for one hiccup:
akka-raft decided to adopt 0-indexed logs, rather than 1-indexed logs as the
paper suggests. This creates a problem:
the initial value of prevLogIndex
is ambiguous:
- followers can’t distinguish between an
AppendEntries
for an empty log (prevLogIndex
== 0)
- an
AppendEntries
for the leader’s 1st command (prevLogIndex
== 0), and
- an
AppendEntries
for the leader’s 2nd command (prevLogIndex
== 1 - 1 == 0).
The last two cases need to be distinguishable.
Otherwise followers won’t be able to reject inconsistent logs. This corner would have
been hard to anticipate; at first glance it seems fine to adopt the convention
that logs should be 0-indexed instead of 1-indexed.
raft-42: Quorum computed incorrectly.
We also found a fuzz test that ended in a violation of the ‘Leader Completeness’ invariant, i.e. a newly elected leader
had a log that was irrecoverably inconsistent with the logs of previous leaders.
Leaders are supposed to
commit log entries to their state machine when they knows that a quorum (N/2+1) of
the processes in the cluster have that entry replicated in their logs.
akka-raft had a bug where it computed the highest replicated log index incorrectly.
First it sorted the values of matchIndex
(which denote the highest log entry index
known to be replicated on each peer). But rather than computing the median
(or more specifically, the N/2+1’st) of the sorted entries, it computed the mode of the sorted
entries. This caused the leader to commit entries too early, before a quorum
actually had that entry replicated. In our fuzz test, message delays allowed another leader to become elected, but it did not have all
committed entries in its log due to the previously leader committing too soon.
raft-62: Crash-recovery not yet supported, yet inadvertently triggerable.
Through fuzz testing I found one other case where two leaders became elected in
the same term.
The Raft protocol assumes a crash-recovery failure model – that is, it allows
for the possibility that crashed nodes will rejoin the cluster (with non-volatile state
intact).
The current version of akka-raft does does not write anything to disk
(although the akka-raft developers intend to support persistence soon).
That’s actually fine – it just means that akka-raft currently assumes a crash-stop failure
model, where crashed nodes are never allowed to come back.
The Akka runtime, however, has a default
behavior that doesn’t play nicely
with akka-raft’s crash-stop failure assumption: it automatically restarts any process that throws an exception.
When the process restarts, all its state is reinitialized.
If for any reason, a process throws an exception after it has voted for
another candidate, it will later rejoin the cluster, having forgotten who it had
voted for (since all state is volatile). Similar to raft-56,
this caused two leaders to be elected in our fuzz test.
raft-66: Followers unnecessarily overwrite log entries.
The last issue I found is only possible to trigger if the underlying
transport protocol is UDP, since it requires reorderings of messages between
the same source, destination pair. The akka-raft developers say they don’t currently
support UDP, but it’s on their radar.
The invariant violation here was a violation of the ‘Leader Completeness’ safety property, where a leader is
elected that doesn’t have all of the needed log entries.
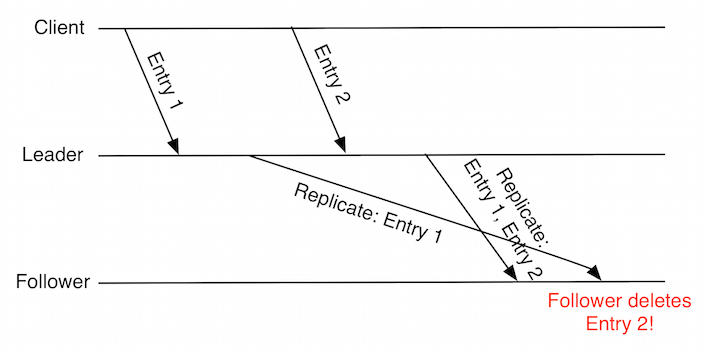
Leaders replicate uncommitted ClientCommands
to the rest of the cluster in batches.
Suppose a follower with an empty log receives an AppendEntries
containing
two entries. The follower appends these to its log.
Then the follower subsequently receives an AppendEntries
containing only the
first of the previous two entries. (This message was delayed, as shown in the
Lamport Time Diagram). The follower will inadvertently delete the second entry from its log.
This is not just a performance issue: after receiving an ACK from the follower, the leader is under the impression that the
follower has two entries in its log. The leader may have decided to commit both
entries if a quorum was achieved. If another leader becomes elected, it will not necessarily have
both committed entries in its log as it should.
Conclusion
The wide variety of bugs we found gets me really excited about how useful our
fuzzing and minimization tool is turning out to be. The development toolchain for
distributed systems is seriously deficient, and I hope that testing techniques like
this see more widespread adoption in the future.
I left many details of our approach out of this post for brevity’s sake,
particularly a description of my favorite part: how DEMi minimizes the faulty executions
it finds to make them easier to understand. Check
out our paper draft for more details!
[1] RPC layer interposition does come with a drawback: we’re tied to a
particular RPC library. It would be tedious for us to adapt
our interposition to the impressive range of systems Jepsen has been applied
to.
[2] How we perform this minimization is
outside the scope of this blog post. Minimization is, in my opinion, the most
interesting part of what we’re doing here. Check out our
paper draft for more
information!